Artificial intelligence for the autonomous trams
Autonomous train project in partner with Albtal-Verkehrs-Gesellschaft in Karlsruhe is one of the most complete projects seen in terms of challenges (time, complexity, innovation, result, etc.) but also diversity in terms of technology. But before focusing on the technology and the solution, it is important to explain the operational use case which leads to the technical solution.
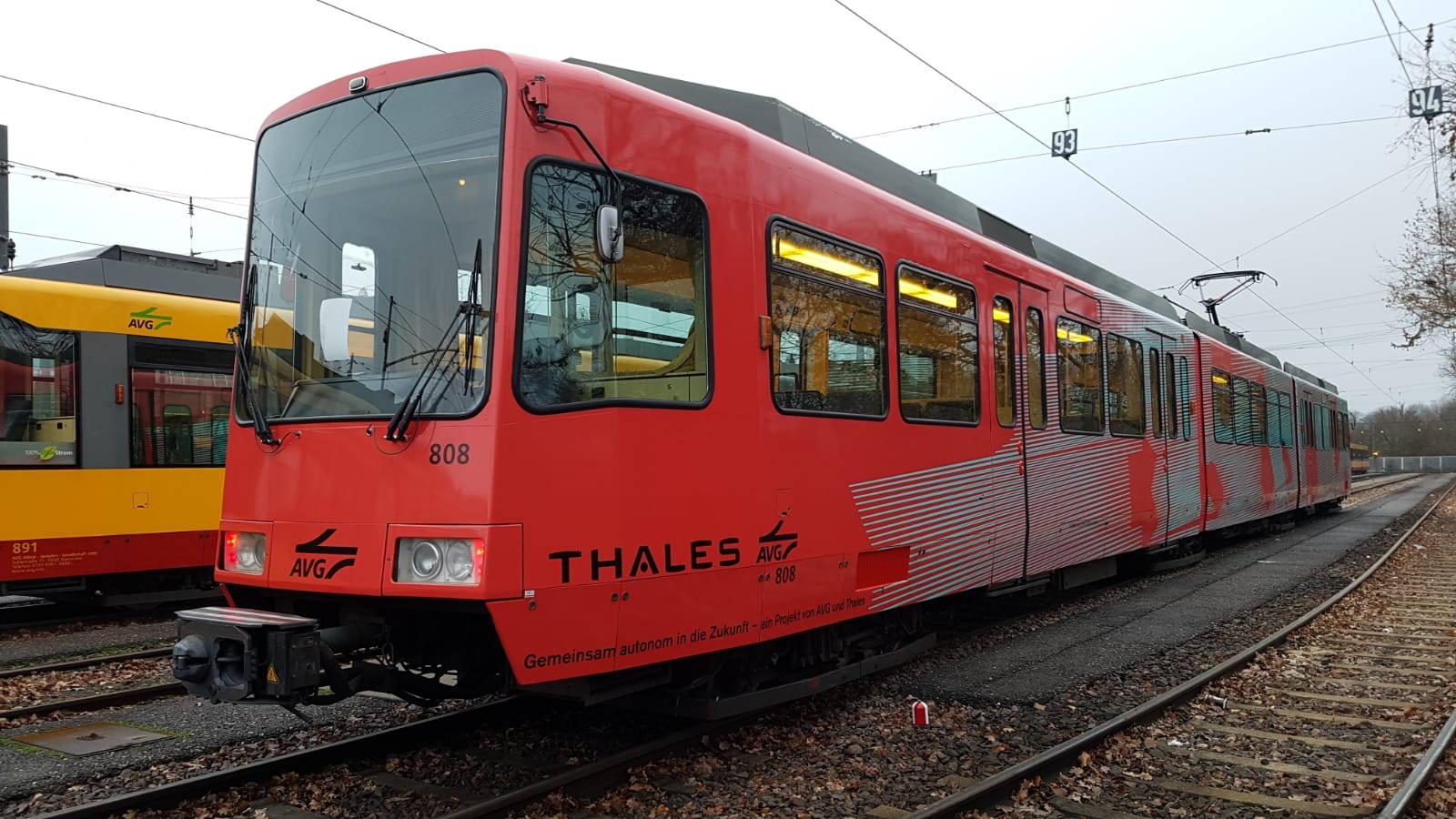
Initially, we focused on the optimization of current processes and therefore the first phase was targeting a use case related to the depot management. First of all today, the driver needs to park the train in the depot at night after the shift, where in peak times the trains queue up and a driver can lose up to 45 minutes. The second tasks are the nightly routines such as maintenance and cleaning performed by the maintenance guys where they lose a lot of time by shunting the trains. These different tasks are automatically and continuously impacting the performance of the different staff.
There would be a huge process optimization and efficiency increase if the train would park themselves autonomously and perform later night the routine jobs after a full day operation with grade of automation level 3 (GoA3). This would avoid using high skilled maintenance engineers for shunting activities and let them focus on the advance tasks they need to perform for maintaining the trains.
In Mid-December 2018, the first trials in GoA3 in the Karlsruhe depot were successfully performed confirming Thales capabilities and technologies being real assets in order to deliver quicker and to help our customer to improve their railway network.
Automatic versus autonomous
The automatic metros or the sky trains in airports are famous for their driverless capabilities. However these solutions are automatic trains and not autonomous trains. Automatic trains are performing in a closed environment, therefore no external events are disturbing the operation. Contrarily to automatic trains, autonomous trains are operating in an open or semi-open environment and unplanned events may occur; the train needs to react to this kind of unknown situations. This is where we come to the question of how the autonomous train can continuously be aware of the surrounding situation? The answer is to make the trains more intelligent and to equip them with the appropriate senses to enable real time awareness.
There are different types of senses for railway: near range, mid-range and/or long-range sensors. These types of sensors are selected according to different parameters, but the main factor remains the speed of the train which indicates the braking distance for the train to stop and which results in the sensor’s performance of detection range. In the mid and long range, we can see the limit of the automotive industry sensors. This is where other industries like defence and avionics can play a significant role for such solutions.
Thales group is positioned as a unique leader in various technologies provided by the diversity of divisions and products, for example, sensors and obstacle detection from our defence and optronic business units, safe and precise satellite positioning from our space and avionics division as well as cyber security from the security sector. Of course all these technologies apply to the state-of-the-art safety from the ground transportations sector. This technology diversity helps Thales to get quicker to the point and reach a minimum viable product rapidly with a high end and premium user experience, by applying new methods of development and agility boosted by the Thales digital factory. The Robot train (RailBot™) is one of the most safe advance technologies in the world of mobility.
Detection, Monitor and Identify
One of the big challenges in railway is to find the right balance between safety and availability, but in fact there is none. If the train is stopping all the time in case of a false detection, the availability of the railway service would be in danger.
One very important aspect in the autonomous train is to bring a reliable vision capability to the train; this capability is here to safely detect obstacles in front of the train but also to monitor the environment around the track.
In order to have an efficient solution we need to differentiate between the different levels of visions; the first one is detection, followed by recognition and finally the obstacle identification.
It is crucial to differentiate between just detecting, recognizing and identifying an obstacle. The first one helps to anticipate and then focus on that specific area in order to classify the category of the obstacle.
If for example there is a detected object but this object is not harmful to the train (e.g. plastic bag, box, etc.), the reaction of the train will be different than if there is a tree or a truck blocking the route of the train.
Here, Artificial Intelligence comes into the game, where deep learning plays a huge role to learn about the type of obstacles the train is facing, and how dangerous it is according to the speed, weather condition and layout of the track.
In this use case, there are three different levels of artificial intelligence:
- the first one is to detect the type of obstacle (car, truck, person, staff, tree, bicycle, etc…),
- the second to detect the intention of the object, if it is a static object or a moving object, entering or leaving the track.
- The third level deals more with the decision and action the train should take (emergency brake, deceleration, acceleration, honking, etc…).
These with AI advanced sensors will bring the train industry to the next level of operational availability, as RailBot™ will see much further obstacles, in bad weather conditions, as well in low light conditions, and also will anticipate situations which could not be done before.
There is a conviction that with autonomy we can help the railway to have a better position in global mobility, and therefore a better and more flexible mobility for the passengers.