Trusted AI: a strategic challenge
Making the right decision at a critical moment can be a matter of life or death. That’s why Thales, which serves sensitive industries such as aerospace, space and defence, is taking steps to build trusted artificial intelligence (AI) technologies that keep humans at the centre of the decision-making process. As global leaders in the field, the Group’s R&D teams are rising to the challenge of developing AI systems that work as intended, can explain how they reached their conclusions and are certifiable for use in critical situations.
David Sadek, VP, Research, Technology & Innovation at Thales, specifically in charge of Artificial Intelligence & Information Processing, tells us more.
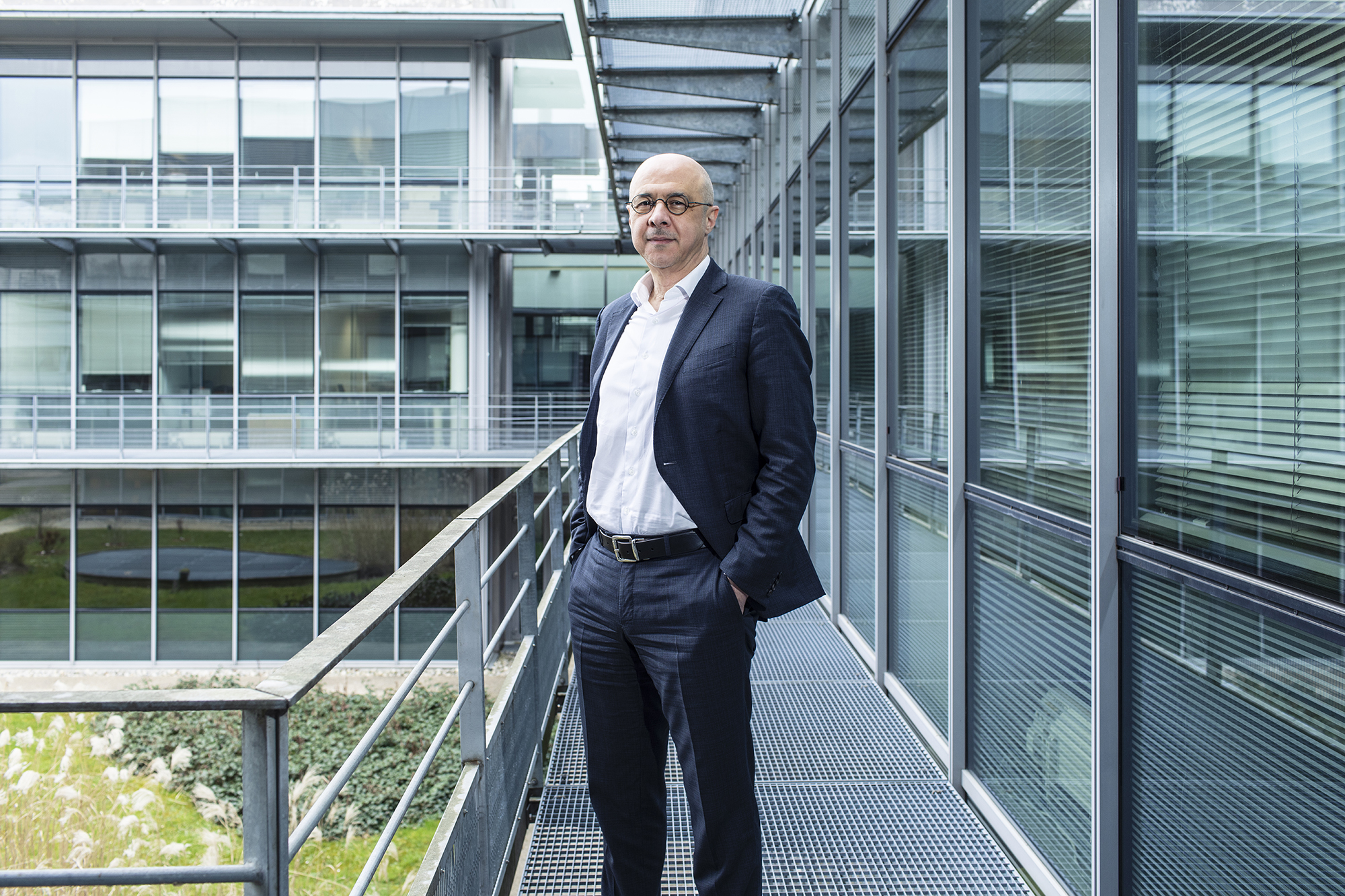
Thales is investing heavily in the development of AI-based systems. But how can we be certain that we can trust AI in aerospace, defence and other critical industries?
Thales operates in critical sectors and industries, where the stakes can be incredibly high. When it comes to developing digital systems for these markets, especially AI-based systems, the requirements are particularly tight. If the step-counter app on your smartphone produces an inaccurate reading because the algorithm is only approximate, it’s hardly a big deal. But for the systems that get planes safely on and off the ground – and one in three aircraft worldwide uses Thales technologies for just that purpose – the standard is entirely different. For critical systems like these, where lives are at stake, trusted AI is the only option. And merely saying that an AI system can be trusted isn’t enough. You have to prove it. Thales operates in a heavily regulated environment with strict controls. We can’t simply do as we please: the AI technologies we build into our systems have to meet stringent requirements, and in some cases, they need to obtain certification before they can be used in real-world applications. Our approach to trusted AI is based on four key principles: validity, security, explainability and responsibility.
These principles might sound a little abstract. How do these properties of trusted AI translate into the day-to-day work of Thales’s R&D teams?
Our people working on AI systems are tackling some of the biggest challenges in science and technology today. When you’re developing an AI-based system, your first priority is to demonstrate its validity. In other words, you have to make sure it works as intended – nothing more, nothing less. There’s no question of installing AI technology in an aircraft or other critical system unless it’s been validated. We do this using mathematical tools, methods and processes, all of which takes time and resources. To take one example, there’s still no sure-fire way to validate a machine learning system. How do you prove that such a system will do exactly what it’s expected to do in its operational design domain (ODD), or the precise conditions in which it was designed to function? If the algorithm under-calculates the length of runway needed to land an Airbus, that’s a problem. But if it over-calculates the length, we could live with that. This raises the question of what’s acceptable. And things could become even more complex with the emergence of continuous learning AI – systems that learn from the data they collect and improve their performance as they go along. Therein lies the challenge – proving that these systems will remain valid after they’ve entered service, even though they keep learning. But we also need to strike a balance, or we risk losing those nice surprises that AI throws our way every now and then. For instance, when the AlphaGo algorithm beat the world Go champion in 2017, it came up with new strategies that had never occurred to even the brightest human mind. In avionics, there’s no room for error: the maximum allowable probability for a hazardous failure condition is 10-9 per flight hour. It’s a tiny number, but that’s the standard we have to meet. In fact, we’re aiming for a probability of 10-12 for our systems.
Protecting AI-based systems from cyber risks is another huge challenge. How do we eliminate vulnerabilities and make sure these systems can withstand cyberattacks and other threats?
Cybersecurity is, of course, a top priority. It’s important to demonstrate that AI systems are robust enough to withstand cyberattacks and other malicious acts. These days, the relationship between security and AI works both ways: as well as building cybersecure AI-based systems, we’re also using AI to develop cybersecurity algorithms. Machine learning algorithms, for instance, can scan an event log and pick up weak signals that may point to an impending cyberattack. We’ve seen examples of attackers exploiting flaws to trick artificial neural network-based systems into misbehaving, such as by using online software to alter images in ways that are barely perceptible to the human eye or, as in one recent case, placing stickers on street signs to fool self-driving cars. When it comes to cyberfraud and other forms of malicious activity, AI technology is progressing at the same pace as the threats. Thales has put together a team of ethical hackers, based at the ThereSIS laboratory in Palaiseau, to make sure our AI systems are resilient to cyberattacks. They identify vulnerabilities by subjecting algorithms, and neural network architectures in particular, to a battery of “crash tests”, then recommend countermeasures to make our applications as robust as possible. The team is currently developing a suite of tools known as the “Battle Box”. This “crash test” laboratory is an integral part of the certification process for our AI-based systems.
The algorithms built into critical systems make decisions automatically. We expect AI to get these decisions right, but how do we understand and explain why they were made?
Explainability is a key priority. It isn’t a new concept, but it’s come to the fore again with the emergence of artificial neural networks. In short, it’s about explaining why a system made a particular decision, and some algorithms are so complex that nobody – not even experts – can explain how they work! We know why a particular decision was reached, but we don't know how. This is what’s known in the industry as the “black box” phenomenon: we can explain an output without understanding every detail of the process that caused it. On a similar note, it’s important to be able to demonstrate that a system has actually learned what we wanted it to learn. Explainability is one of the main focuses of our research at Thales, in particular at the Sinclair lab (Saclay Industrial Collaborative Laboratory for Artificial Intelligence Research), a joint R&D unit set up with EDF and Total to work on this very topic, as well as on simulation and reinforcement learning. Mislearning can be a real problem for AI technologies. I recall one case where a system was taught to recognise lions in the savannah. One day, it was shown a picture of a cow in the savannah and identified it as a lion. The system had learned to recognise the savannah – the background – but nothing else. In other words, it had mislearned. To take another example, towards the end of the Cold War, the US military was using an AI-based system to spot Soviet tanks in images. The system worked well until, one day, it identified “Soviet tanks” in a set of blurry holiday snaps. Using reverse engineering techniques, the team came to realise that the system was erroneously marking all blurry images as containing tanks. The issue of explainability also applies to symbolic, or rules-based, AI: when systems rely on a spaghetti-like jumble of rules to make deductions, it becomes almost impossible to determine the process by which inputs are converted into outputs.
What is the answer to this problem of mislearning?
At Thales, our aim is to achieve real-time explainability by developing “self-explaining” AI – systems that can account for their behaviour on the fly. Take a digital co-pilot system on board an aircraft, for example, and imagine it tells the human pilot to turn 45 degrees in 30 miles. The pilot needs to be able to ask “Why?” especially if they were planning a quite different course of action. And the system needs to answer in way that the pilot can understand. It needs to say “because there’s a (military) threat ahead or a storm brewing” – not “because neural network layer 3 was 30% activated”. What qualifies as an explanation? Should it be appropriate to the context and to the pilot’s cognitive burden? Should it be provided in real time in the primary sense of the term, i.e. before the environment changes? If the pilot has five seconds to make a decision, the AI system cannot take more than five seconds to respond. These are crucially important questions with significant implications for how the explanation is provided: using the right channel of communication, keeping the language level appropriate and the message short enough, and tailoring all of this to the receiver’s mental state and stress level. In other words, the aim is to use carefully chosen key words without going into unnecessary details that could cloud the message.
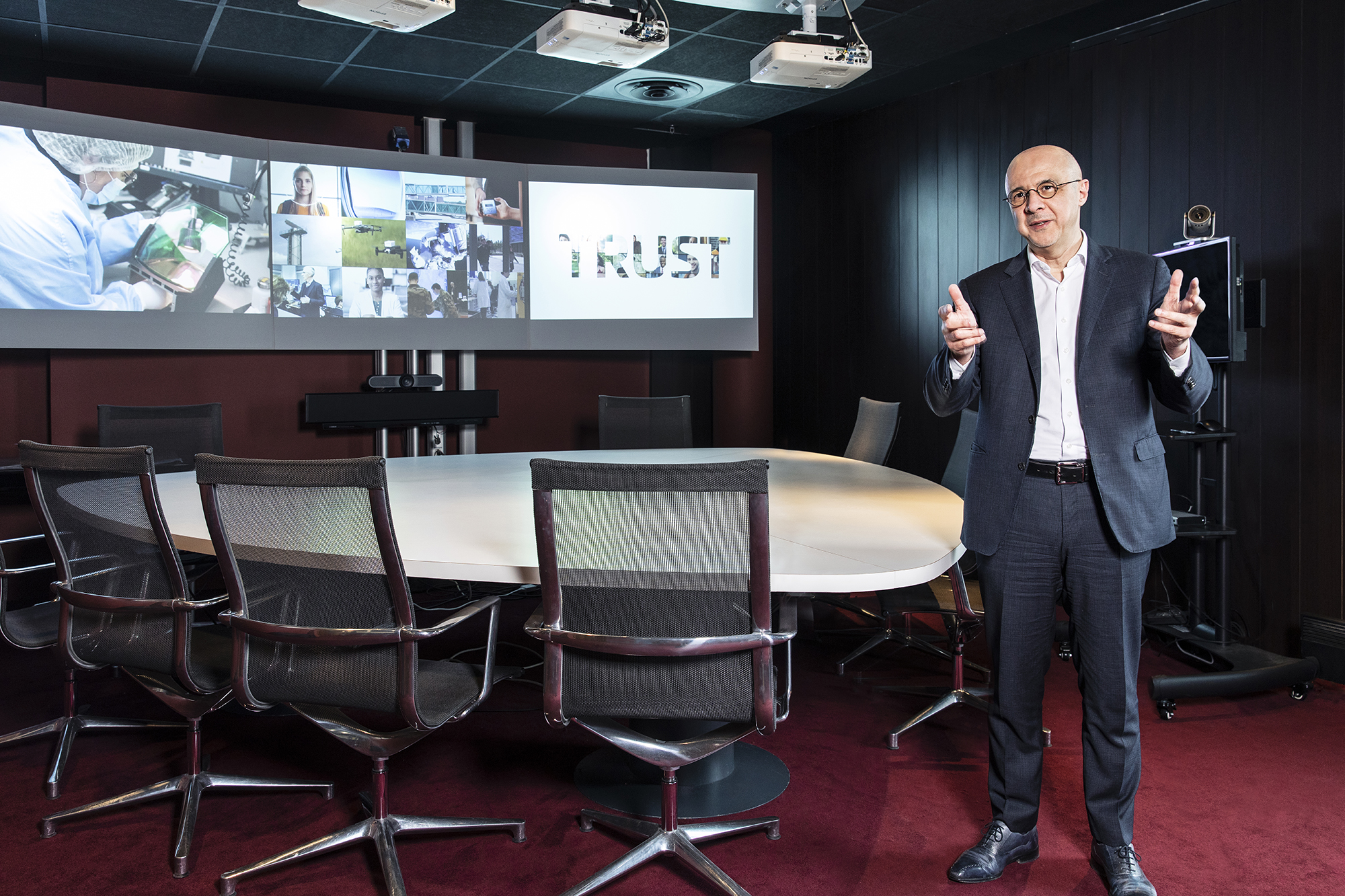
So it's crucially important not to overlook the human dimension of AI-based systems.
Exactly. At Thales, we’ve set a high bar for ourselves on this point. Most of our systems are designed around decision-making chains and therefore require human input. Human-machine dialogue is a matter of strategic importance. Our challenge is to develop and deploy AI systems that support intuitive human-machine interaction and dialogue – in other words, a conversation that extends beyond mere questions and answers. You could say that this is the holy grail of AI. There’s a good reason why the Turing test (designed to measure a machine’s ability to exhibit human-like intelligence, ed.) was built around dialogue. Until now, explainability has been the preserve of experts, but we absolutely have to bring users into the loop, so they can tell us whether a given explanation works for them. It’s a technically complex subject, but we mustn't lose sight of how AI systems interact with human beings. Indeed, our commitment to keeping humans in control of AI is set out in black and white in our Digital Ethics Charter. And anyone who’s read Isaac Asimov will know that his Three Laws of Robotics*, which govern the interaction between robots and humans, are as relevant today as they were in 1942. Today, our approach to AI is fundamentally guided by the notion of responsibility – designing systems and technologies that adhere to legal, regulatory and ethical frameworks. Going forward, documenting, modelling and implementing these rules should be a priority.
*1. A robot may not injure a human being or, through inaction, allow a human being to come to harm. 2. A robot must obey the orders given it by human beings except where such orders would conflict with the First Law. 3. A robot must protect its own existence as long as such protection does not conflict with the First or Second Law.
What sets Thales apart from other players in the AI space?
At Thales, we firmly believe that hybrid AI offers the best way forward, since it combines the benefits of data-based systems and model- and knowledge-based algorithms. We were also among the first companies to push back against indiscriminate data collection and to advocate for collecting only the data that's needed. In doing so, we rejected the concept of “big data” – a lazy approach to AI development – and adopted the principle of “smart data”, which allows us to keep the amount of data we use to a minimum while applying techniques such as transfer learning (using knowledge gained while solving one problem to solve a different but related problem, ed.) to make our algorithms smarter and more human-like in their behaviour. This frugal approach to data is consistent with the advances we’re making in “neuromorphic” processors, which are far less energy-hungry than conventional artificial neutral networks.
So you’re factoring environmental concerns into the way you develop your AI systems?
Indeed we are. This is precisely the thinking behind my proposals for the Green AI and AI for Green initiatives, which are key aspects of our current work. AI technology is incredibly energy-intensive. At Thales, we’re employing frugal learning methods – which are proportionate in the use of data – to make our AI systems as environmentally responsible as possible. But AI can actually help to reduce carbon emissions too. For instance, Thales is leading the way on developing algorithms that adjust aircraft trajectories to limit the production of environmentally harmful contrails. We’re also exploring cutting-edge AI technologies such as reinforcement learning (where a machine learns not from training data but through trial and error, ed.), which is an incredibly promising field. And we’re using AI-based systems that factor in the wake vortex effect to limit how long aircraft spend in the air on final approach and reduce their carbon emissions. Internally, we’re planning to launch a call for ideas on how we could leverage AI to reduce the Group’s carbon footprint. Ethical and environmental considerations should be perceived not as constraints but as opportunities for value creation and differentiation. In our view, when you have a choice between solutions with comparable functionality, it’s always best to go for the greenest and most ethical option.
What avenues of innovation are you currently exploring at Thales? And what would you say to a young engineer interested in working in AI?
Much of our current work focuses on AI engineering – on building the tools, methods and processes we need to develop these systems at scale. We’re also working on distributed AI, which involves decentralising decision-making through multi-agent systems (MAS). The Thales School of AI* was set up specifically to provide the Group with a pool of fully trained AI experts. To a young engineer interested in working in AI at Thales, I’d say that a career with us is a chance to take on some of the most exciting challenges in science and technology today. And I’d stress that AI isn’t limited to specific use cases –these technologies have applications in fields across a whole spectrum of areas: aerospace, space, defence, digital identity and security, to name but a few. Given that science is a constant search for simple, universal truths, I’d describe the development of trusted AI as a perfect opportunity to harness the power of technology to build a better world for all.